People
Maggie Johnson
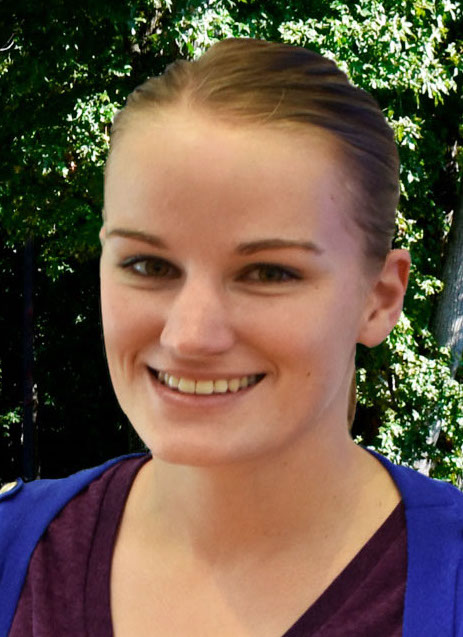
Maggie Johnson
Biographical Sketch
Maggie Johnson is a data scientist at the Jet Propulsion Laboratory. She received her PhD in Statistics from Iowa State University in 2017. She has an MS in statistics from Iowa State University and a BS in Mathematics from the University of Minnesota, Twin Cities. Her broad research interests are in developing statistical methods for applications in the environmental and Earth sciences. She has particular expertise in spatial and spatiotemporal statistics for massive remote sensing data, statistical data fusion, Bayesian hierarchical modeling, and uncertainty quantification.Her research has supported multiple NASA Earth Science satellite missions, including developing novel, computationally-scalable data fusion methods for the ECOsystem Spaceborne Thermal Radiometer Experiment on Space Station (ECOSTRESS) and Surface Biology and Geology (SBG) missions, and leading uncertainty quantification efforts for the Aura Microwave Limb Sounder (MLS). Additionally, she has led multiple JPL research and development grants developing statistical data fusion methods for remotely sensed imagery.
Interest areas: remote sensing data fusion, spatial and spatiotemporal statistics, state-space models, uncertainty quantification
Software
STARS (Spatial Timeseries for Automated high-Resolution multi-Sensor data fusion)Projects
- Microwave Limb Sounder (MLS)
- ECOSTRESS Evapotranspiration Analysis
Selected Publications
Simafranca, N., Willoughby, B., O'Neil, E., Farr, S., Reich, B. J. and others including Johnson, M. (2024). “Modelling wildland fire burn severity in California using a spatial Super Learner approach,” Environmental and Ecological Statistics.
Halverson, G., Cawse-Nicholson, K., and Johnson, M. (2023). “ECOSTRESS Collection 2 Level 1-2-3-4 Gridded and Tiled Products User Guide,” Document.
Hook, S., Cawse-Nicholson, K., Halverson, G., Hatch, B., Johnson, M. C. and others (2023). “ECOSTRESS Level-3 Evapotranspiration L3 (JET) Algorithm Theoretical Basis Document,” Document.
Nimrod Carmon, Alexander Berk, Niklas Bohn, Phillip G. Brodrick, Jeff Dozier and others including Margaret Johnson (2023). “Shape from spectra,” Remote Sensing of Environment, 288, p. 113497.
Johnson, M., Reich, B. J., and Gray, J. (2021). “Multisensor Fusion of Remotely Sensed Vegetation Indices using Multivariate Space-Time Dynamic Linear Models,” Journal of the Royal Statistical Society, Series C, 70(3), pp. 793-812.
Cawse-Nicholson, K., Braverman, A., Kang, E., Li, M., Johnson, M. and others (2020). “Sensitivity and uncertainty quantification for the ECOSTRESS evapotranspiration algorithm – DisALEXI,” International Journal of Applied Earth Observation and Geoinformation, 89, p. 102088. Document (link to DOI).
von Clarmann, T., Degenstein, D. A., Livesey, N. J., Bender, S., Braverman, A. and others including Johnson, M. C. (2020). “Overview: Estimating and reporting uncertainties in remotely sensed atmospheric composition and temperature,” Atmospheric Measurement Techniques, 13(8), pp. 4393–4436.
Johnson, M., Caragea, P.C., Meiring, W., Jeganathan, C., and Atkinson, P.M. (2019). “Bayesian Dynamic Linear Models for Estimation of Phenological Events from Remote Sensing Data,” JABES, 24(1). Document.
Guan, Y., Johnson, M., Katzfuss, M., Mannshardt-Hawk, E., Messier, K. P. and others (2019). “Fine-scale spatiotemporal air pollution analysis using mobile monitors on Google Street View vehicles,” JASA Applications & Case Studies, 115(531), pp. 1111-1124.